Systematic reviews are a cornerstone of evidence-based medicine, but the process of conducting them can be time-consuming and labor-intensive. Covidence is constantly innovating to streamline the process for researchers.
In this blog post, we will explore how Covidence is harnessing the power of Machine Learning (ML) to enhance the systematic review workflow. We’ll start with our existing features, including the RCT Classifier, Remove references reporting on non-RCTs before screening, and Relevance Sorting in Screening, highlighting how they save valuable time and effort. Additionally, we’ll provide insights into our future developments aimed at further automating and optimizing the systematic review process.
Existing machine learning features in Covidence
1. RCT Classifier
Identifying randomized controlled trials (RCTs) is crucial for interventional systematic reviews, as they often provide the highest quality evidence. Developed by the Cochrane collaboration, the RCT classifier is integrated into Covidence and uses ML to help you quickly and accurately identify RCTs in your search results. Trained on a massive dataset of over 280,000 records, this classifier boasts an impressive sensitivity of over 99.5% (Thomas et al, 2021). This means it’s unlikely to miss an RCT in your search results, saving you valuable time that would have been spent manually screening articles.
2. Remove references reporting on non-RCTs before screening
Building on the RCT Classifier, this additional feature removes references reporting on non-RCTs from the search results before screening. This intelligent filtering mechanism significantly reduces the initial screening workload, allowing researchers to focus their efforts on the most relevant studies.
3. Relevance sorting in screening
Covidence’s Relevance Sorting feature intelligently orders studies, based on previous screening decisions. This is achieved by using an active learning model that predicts the likelihood of the inclusion of the remaining references based on the user’s past screening decisions. The system leverages the titles and abstracts of screened studies to prioritize those likely to be relevant, thus facilitating a more efficient screening process. It helps streamline the screening process, especially in teams with multiple reviewers working on different stages of the review.
Future machine learning developments
1. Automatic removal of irrelevant references based on PICOs
Covidence is developing a feature to automatically remove references that do not align with the specified inclusion criteria detailed in the study’s Population, Intervention, Comparison, Outcome (PICO) framework. This feature aims to use ML to evaluate the relevance of articles before the screening phase begins, reducing the initial number of articles for manual review.
2. Exploration in Data Extraction and Quality Assessment
Covidence is actively exploring the potential of ML to streamline the data extraction and quality assessment phases of systematic reviews. These critical stages involve gathering and evaluating data from selected studies, and enhancements in this area could further reduce manual workload while maintaining the integrity of the review process.
3. Feedback from the Systematic Review community
During the Covidence ML webinar, members of the systematic review community highlighted areas for potential ML application.
They especially emphasized the need for automation in data extraction and quality assessment to further streamline systematic reviews.
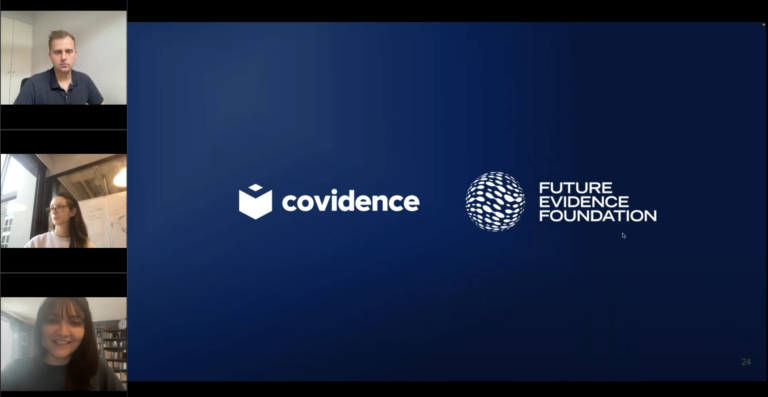
Notably, a significant number of participants expressed a desire for automation at the search stage—a phase Covidence does not currently support but may consider for future development. This feedback is instrumental in guiding Covidence’s evolution to meet the evolving needs of the research community efficiently.
Conclusion
Covidence’s integration of ML features like the RCT Classifier, remove references reporting on non-RCTs before screening, and Relevance Sorting, along with its ongoing development of new tools, are transforming the landscape of systematic review processes. These advancements enhance efficiency, accuracy, and reduce bias, emphasizing Covidence’s commitment to supporting high-quality, evidence-based medicine. As we continue to evolve with input from the research community, Covidence solidifies its position as an indispensable tool for researchers worldwide.
To get started, sign in here.
Or if you’re new to Covidence, click here to try it for free!