Keep up to date on new features and bug fixes on Covidence.
At Covidence, we are dedicated to providing our customers with the best service and systematic review tool possible. To ensure this, we are constantly reinvesting in our product to stay up-to-date with the latest technology. One such technology is machine learning, also known as active learning. This rapidly growing technology field is revolutionising how teams review studies, and we have now implemented it within Covidence.
Highlights:
- Sorting studies in title and abstract screening using machine learning
- Import effect estimates into RevMan 5
Move studies through to full-text review sooner using machine learning study sorting.
Machine learning (active learning) is now helping teams move relevant studies through to full-text review sooner. By utilising machine learning algorithms, teams are able to quickly identify relevant studies and move them through to full-text review much faster than traditional methods.
This technology has the potential to drastically reduce the amount of time and resources needed to complete a study, allowing teams to focus their efforts on more important tasks. With machine learning, teams are now able to work smarter, not harder.
When screening titles and abstracts, teams now have access to a new sort option “Most relevant”.
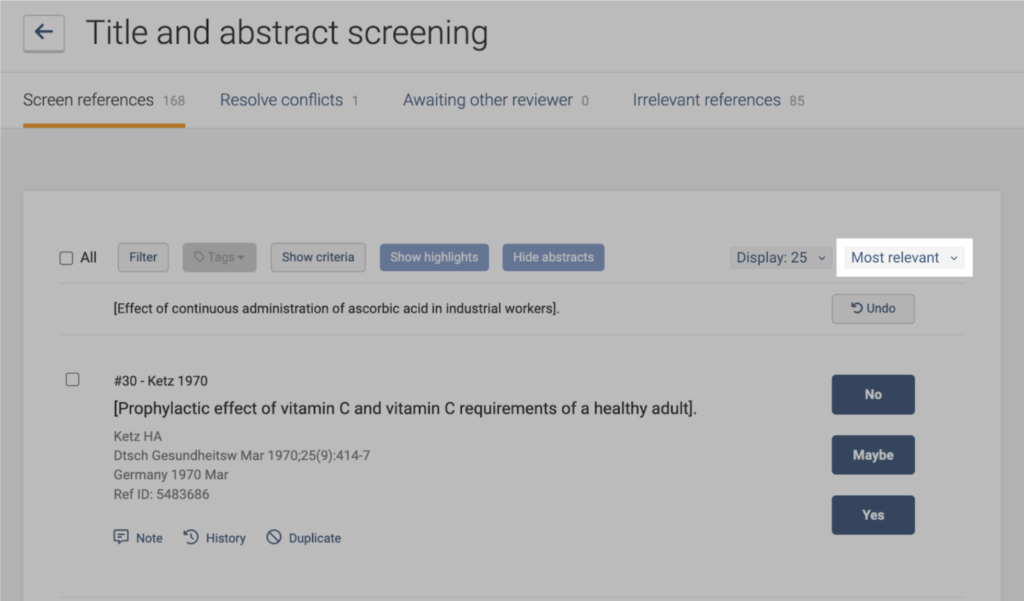
Machine learning accuracy improves the more you use it.
This sorting option utilises machine learning, or active learning, to identify patterns in previous screening behaviour on the review. This allows the system to determine and display the studies that are most likely to be included first. As more studies are screened, the system’s prediction will become stronger and more accurate.
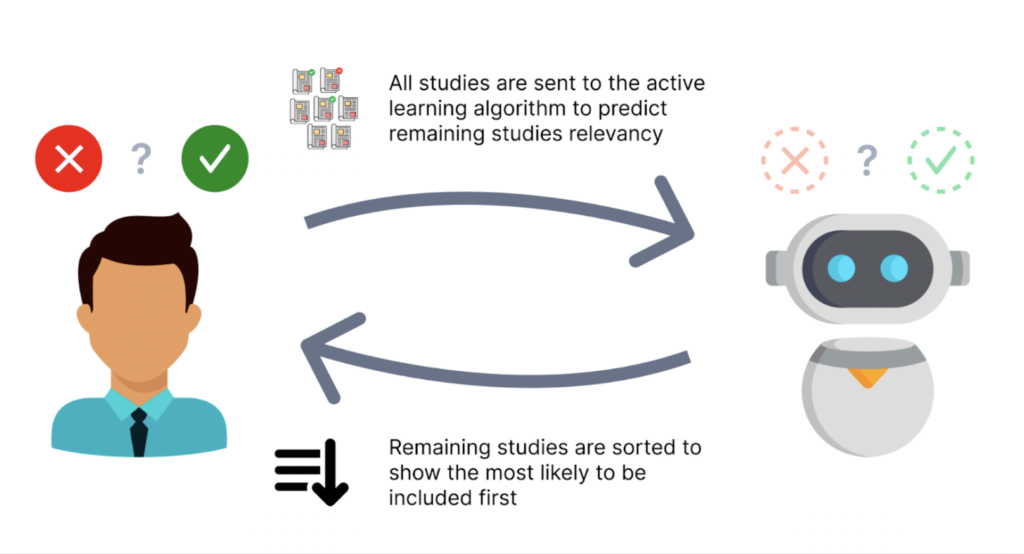
Machine learning uses patterns in your team’s past screening behaviour to determine the relevancy of studies. However, it won’t begin to use the predicted relevancy scores to sort until at least 25 studies have been marked as included or excluded. Of those 25 studies, at least two must be marked as included and two must be marked as excluded before the machine learning algorithm can begin to use the predicted relevancy scores to sort.
Miwa 2014 describes how the active learning algorithm works and the benefits of using it to support screening.
Import effect estimates into RevMan 5
We recently launched a feature that allows you to extract effect estimates (Arm vs Reference arm) using Extraction 1. Now, you can import your extracted data directly into RevMan 5. This feature makes it easier than ever to quickly and accurately import your extracted data into RevMan 5. This new feature is sure to save users time and effort, while ensuring accuracy and reliability.
To download a RevMan 5 file from Covidence, ensure you are using Extraction 1, navigate to the export page, and click on “Export to RevMan 5”.
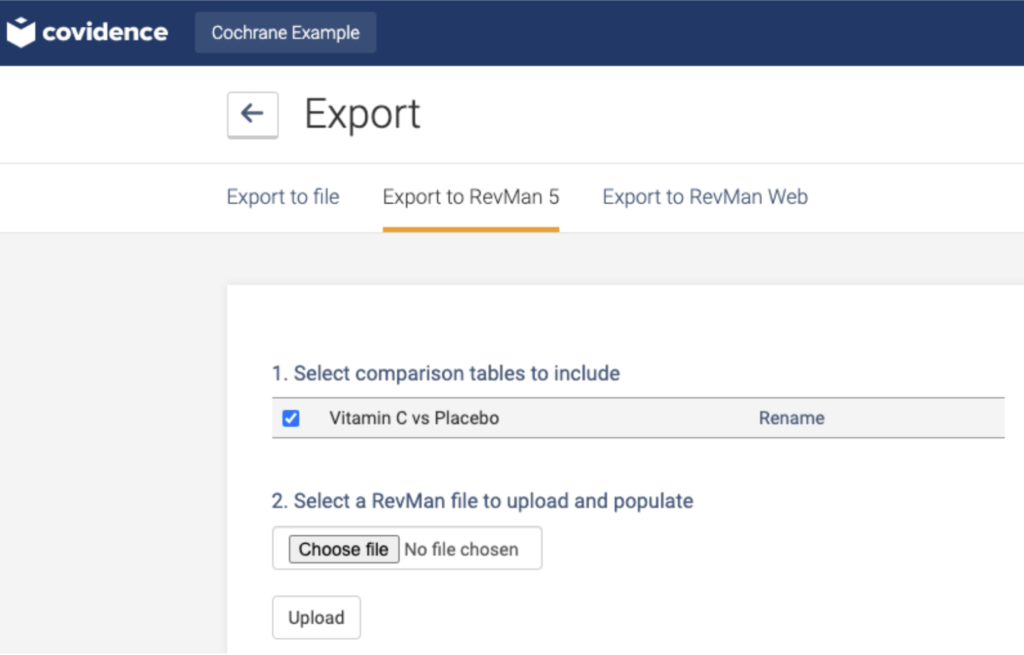