Learn how to complete data extraction with Covidence
With the screening process complete, review teams take the information they need from the studies they have included in the review. This is data extraction: the process of carefully collecting relevant information from studies, and organising it in a way that will enable analysis and synthesis. It’s an interesting stage in any project because it’s the start of adding value and producing work that is more valuable than the sum of its parts.
The list of included studies is the tip of the iceberg. Each study reference represents a huge amount of information. And because you will be interested in some but not all of that information, your approach to data extraction will need to be planned and purposeful to avoid a data deluge.
The data can be split into two types: data from the study and data about the study. An example of data from the study is the dose and timing of the intervention – this informs the evidence synthesis. An example of data about the study is the description of the allocation of patients to the study arms – this informs the assessment of study quality. Both types of data are usually collected by two reviewers who work independently of each other because this helps to minimise the risk of data entry error.
During data extraction reviewers design, test, and populate forms. The forms are then checked and conflicts are investigated and resolved. There is no ‘best’ way to build data extraction form; much depends on the type of review, the type of data, and the specific outcomes of interest. However, the basic process can be organised into five steps:
- Plan 💭
- Pilot 🤔
- Extract 👩💻
- Compare and reach consensus 👀
- Export 📩
We’ll now look at each step in turn and consider some tips for success, whatever the project.
1. Plan
The Cochrane Handbook for Systematic Reviews of Interventions describes data collection as ‘a bridge between what is reported by the original investigators (e.g. in journal articles, abstracts, personal correspondence) and what is ultimately reported by the review authors’. This is shown in figure 1. It is worth taking the time to design and test the data extraction form to make sure that it captures and faithfully represents the source data.
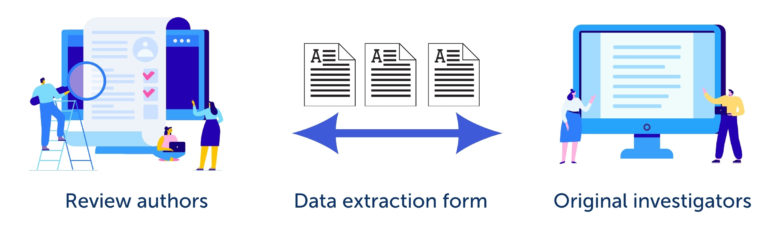
The starting point for designing a data extraction form is the review question and, if you have one, the research plan. The form must be well-structured and easy to use. If items are unclear, there is a risk that the reviewers will make mistakes as they use it. Designing good data extraction forms takes practice. It’s a good idea to take advice from people with experience and to specify in advance the process for resolving conflicts between the reviewers.
Systematic Reviewer Pro-Tip
‘A good data collection form should minimize the need to go back to the source documents’
Cochrane Handbook for Systematic Reviews of Interventions
Covidence helps you to create a logical and well-structured form for your data collection. You can take a template off the peg and customise it to your requirements or, if you prefer, you can start from scratch. This flexibility ensures that the form achieves optimal fit with the data that it is designed to capture.
2. Pilot
The next step is to test-drive the new form on a few studies to check that it works as intended. If the test data come back as per your expectation, the form is performing well. If some test data is wide of the mark, you have a useful opportunity to explore the reasons for this and to improve the form. Is the form capturing everything you need? Is it capturing data that you don’t need? Are any of the questions potentially confusing or open to interpretation? Does it provide data in a form that can be utilized according to your research plan? This testing phase can be repeated until the team is satisfied that the form is fit for purpose.
It’s important to train individual reviewers at the pilot stage and throughout the extraction process to ensure that they understand the requirements and code data in exactly the same way. Discrepancies between reviewers that persist once they are trained could indicate that the form is still not clear enough to gather data reliably.
In Covidence, changes to the data extraction form can be made at any time – not only during the pilot but also once extraction is underway.
3. Extract
With the form ready to go, you’ll now use it to transfer the data safely from the study reports into the review. Although the specifics will be different, each review will likely extract data on the following aspects of the studies:
- Participants
- Interventions
- Outcomes
- Results
These bullets represent data from the study only. Data about the study (for example, risk of bias data) are extracted using a separate form and are not required for all review types (for example, scoping reviews typically do not collect this type of data).
Your review might be interested in some, rather than all, of the interventions in a particular study. There is no need to extract information on the interventions that are out of the scope of the review but it is helpful to provide a complete list for readers who may wish to replicate an intervention. The TIDieR guidelines are a useful reference for reporting interventions.
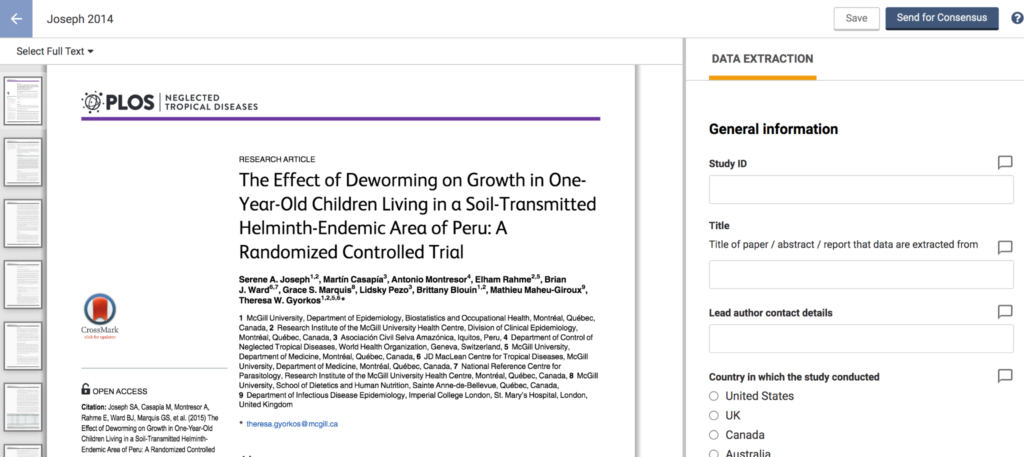
In Covidence you can refer to the full-text of the study on the left of your screen as you complete your data extraction form on the right. Each field in the form has a corresponding comment field. Information saved in comment fields can be useful for the person who produces the consensus data in step 4. Entering study information in the comments can avoid the task of going back to the original report and can also be used to capture information about deviations from the protocol or research plan, which need to be reported in the review.
Covidence has two options for data extraction: version 1.0 and version 2.0. The best fit for your project will depend on factors including the type of review, the type of data, and the type of analysis you plan to do. Review teams can assess the suitability of each tool and switch between them at any time during this step.
4. Compare and reach consensus
The data from the two reviewers must now be compared. Discrepancies must be resolved here and a consensus reached. It is this consensus data that is exported in step 5. If the values in the forms do not match because one reviewer has made a coding error, the error can be corrected here. If the reviewers have reached different judgements, the conflict can be resolved by discussion between the reviewers and, if that doesn’t work, by referral to another member of the team who will make the final decision. It is good practice to specify in advance the process for resolving conflict among the reviewers and to keep a record of these disagreements.
The project dashboard in Covidence flags data that have been extracted but need to be saved as consensus data before the export can begin. The reviewers’ extracted data is collated on one screen for easy comparison. The system also highlights any conflicts and missing values.
The “document” phase may entail some hard-learned lessons, but there is an incredible community of practice of expert searchers and medical librarians present on Twitter (#ExpertSearching, #medlibs) that you can reach out to for help.
Patience is key! Ask questions, reach out for help, and you’ll be an expert searcher in no time at all.
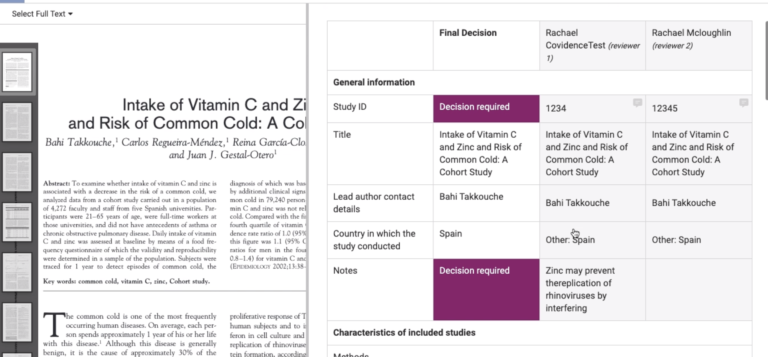
5. Export
With conflicts resolved it’s now time to parcel up the consensus data and download it from Covidence 🎁. Data can be quickly and easily downloaded in the following formats:
- CSV – this facilitates import into statistical programs such as Stata, R, and SPSS.
- If you’re working on a Cochrane Review, Covidence can automatically populate a new RevMan 5 file with the extracted study data and quality assessments.
Conclusion
Data extraction is a critical step in evidence synthesis. Using Covidence for data extraction doesn’t just save time, it also ensures a simple and consistent workflow that minimises the risks of human error and bias. It’s easy to collaborate across the whole team and to keep the project running smoothly. Sign up to Covidence for a free!
References
Li T, Higgins JPT, Deeks JJ (editors). Chapter 5: Collecting data. In: Higgins JPT, Thomas J, Chandler J, Cumpston M, Li T, Page MJ, Welch VA (editors). Cochrane Handbook for Systematic Reviews of Interventions version 6.1 (updated September 2020). Cochrane, 2020.